Onexus.org
is for sale!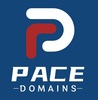
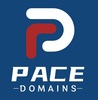
The simple, safe way to buy domain names
No matter what kind of domain you want to buy or lease, we make the transfer simple and safe.
Here’s how it worksSeller's notes about Onexus.org
✨ Grab Onexus.org for Just $1,450 – Deadline April 30! ✨
Act quickly: Onexus.org is yours for the taking at a once-in-a-lifetime price. Make it yours by April 30!
Why It's a Must-Have:
• 🌐 Authority & Trust: .ORG domains stand for respect.
• 🎯 SEO Power: Optimize your search engine presence.
• 📈 Brand Leadership: Carve out a unique niche.
Extra Incentives:
• 📧 Signature Email: your.name@onexus.org sets you apart.
• 🛡️ Defend Your Brand: Secure and steer your online identity.
Smart Choice: Onexus.org represents a growing investment.
Act Fast! The first to hit BUY NOW wins! Seize this opportunity to own a premium domain!